Data scientists from the University of Arkansas (UARK) will develop an Artificial Intelligence (AI) model for biomedical research.
UARK researchers will develop a project of learning modules for biomedical research with the support of the National Institutes of Health (NIH) and Google. The objective of the modules will be to support biomedical researchers in their learning about the importance of machine learning and AI as important tools in research.
For example, biomedical research benefits from the application of machine learning or machine learning by optimizing data analysis for different types of medical and scientific images.
For the development of the project, Justin Zhan, professor at the University of Arkansas, who is the principal investigator of this project, received funds of more than 140 thousand dollars from the National Institute of General Medical Sciences. He also achieved a partnership with Kyle Quinn, professor of biomedical engineering, and Larry Cornett, who heads the Arkansas IDeA Network, a UARK Biomedical Research network.
In this way, the Arkansas IDeA Network will develop case studies for learning modules, using data science and image cores. “New technology is rapidly expanding the number and variety of imaging modalities, for example, that can tell doctors much more about their patients. But this transformation has created challenges, particularly with the storage and management of massive data sets. Furthermore, while the big data revolution transforms biology and medicine into data-driven sciences, traditional education is slow to respond. Addressing this deficiency is part of what we are trying to do,” Zhan explained.
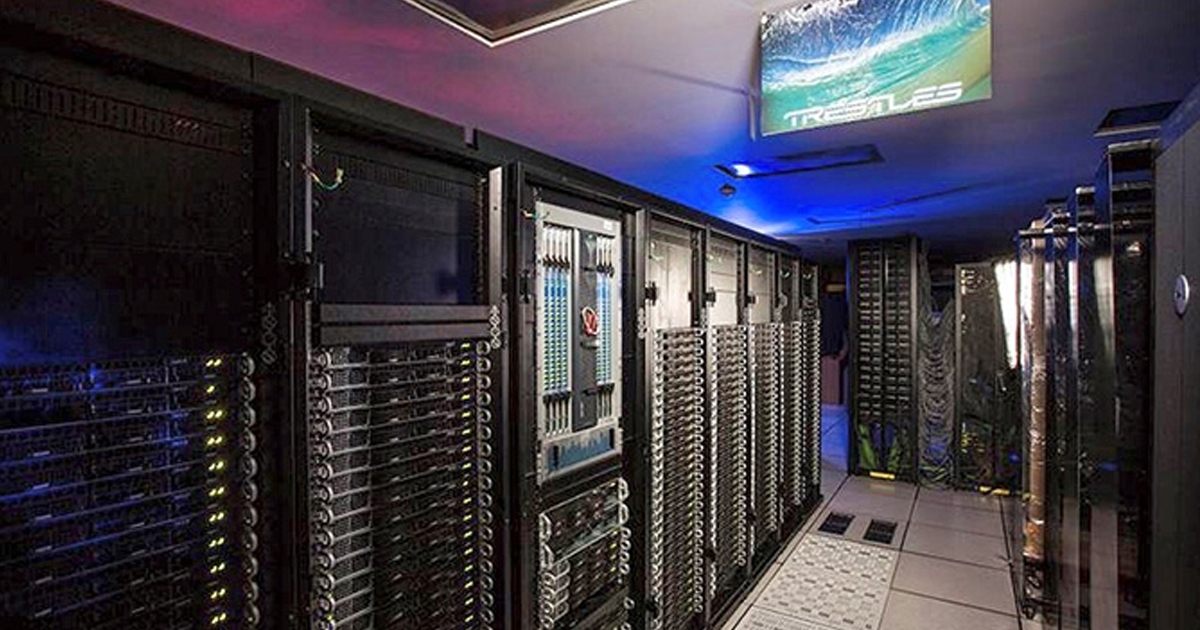
Arkansas researchers will also be supported by software engineers from Google and NIH to address various AI-driven research goals. "The cloud computing modules developed by Zhan's team will help researchers understand how artificial intelligence can be used in biomedical sciences to analyze big data," the university explained in a statement.
Studies related to the identification of features in massive sets of biomedical images could help researchers and clinicians, such as implementing AI in their work on a regular basis.