Substance abuse is prevalent in hospital settings and derives from various behavioral conditions, it is also a problem that is difficult to identify in a comprehensive and reliable manner.
The number of hospital visits related to substance use in the United States increased during the pandemic, and although it is not a recent situation, there are not enough tools to detect these types of conditions. For this reason, researchers in the United States developed an Artificial Intelligence (AI) model to address this situation and published a study that offers details about an algorithm they developed to identify substance abuse through Artificial Intelligence.
The study titled “Development and Multimodal Validation of a Substance Abuse Treatment Referral Algorithm Using Artificial Intelligence (SMART-AI): A Deep Learning Retrospective Study,” aimed to use natural language processing (NLP) and clinical notes in electronic medical records to accurately detect substance abuse.
The model presented in the study was trained on a data set belonging to the Rush University Medical Center in Chicago, with data available from 2017 to December 31, 2019. The key focus for the development of this tool was the notes of ECE performed during the patient's first 24 hours, these were assigned standardized medical vocabulary and were classified with neural network model labels.
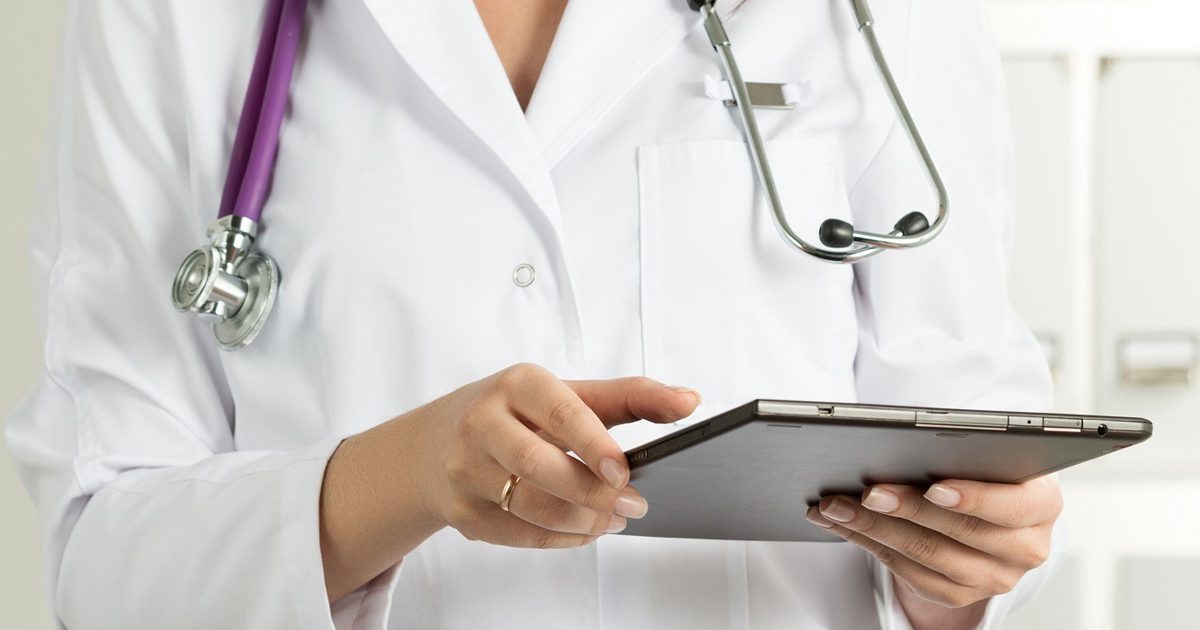
"A machine learning approach treats each word as a feature and does not require subject matter expertise, unlike models that rely on keywords and rule-based systems," the study details.
The model was trained in a cohort of 1,912 patients with 3,5% records of abuse with any type of substance, of which 202 had abuse of more than one type of substance.
“We developed and then temporarily and externally validated an accurate screening tool to identify cases of substance abuse. Our model showed good calibration and face validity. With this model, we offer an automated approach to detect multiple types of substance abuse simultaneously”, explains the study.
You can consult the full study at the following link: https://www.thelancet.com/journals/landig/article/PIIS2589-7500(22)00041-3/fulltext