The Massachusetts Institute of Technology (MIT) has developed a new technique to reduce bias and increase the range of machine learning models.
Artificial Intelligence, machine learning and deep learning models are key aspects for the development of research and studies that require the processing of large amounts of data. However, an imbalance in the data can lead to the creation of models that introduce bias into the research. For this reason, MIT has published a study showing how it was possible to increase fairness in machine learning models.
The article titled: “Is equity just deep metric? Assessing and addressing subgroup gaps in DML”, explains that these models can be corrected. In this way they developed a technique that allows the model to produce fair results regardless of whether it was trained with unbalanced data.
“In machine learning, it is common to blame data for bias in models. But we don't always have balanced data. So we need to come up with methods that actually fix the problem with unbalanced data,” says lead author Natalie Dulero, a graduate student in the Healthy Machine Learning Group at MIT's Computer Science and Artificial Intelligence Laboratory (CSAIL).
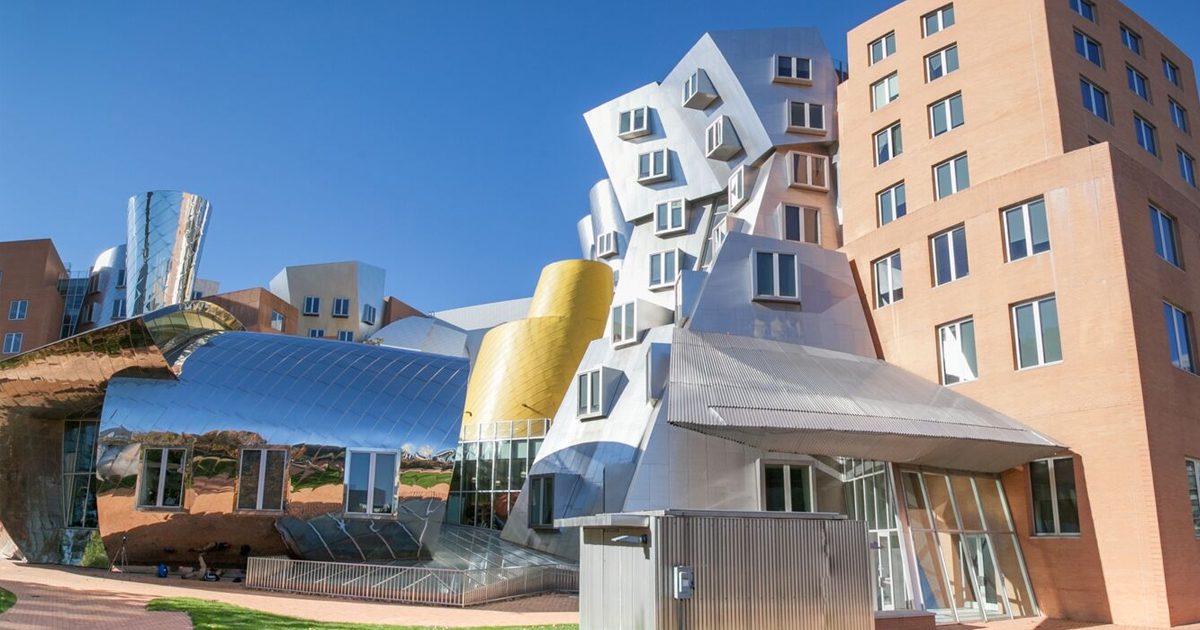
In this sense, the correction of the models can adapt to new data and learn to group new types of information. “We know that data reflects the biases of processes in society. This means that we have to change our approach to design methods that better fit reality,” explains lead author Marzyeh Ghassemi.
Thanks to this type of development it is possible to improve models that have been successful for research. In the case of health, it is important to maintain high standards in deep machine learning models, or any other algorithm, that involves patients.