The growth in the development of Artificial Intelligence (AI) systems and machine learning models applied in clinical settings has also created challenges in their adoption.
Researchers have developed qualitative analyzes through interviews, to measure the difficulties in adopting machine learning models and also the perception of doctors about these models. In this regard, despite a lack of specialized understanding of machine learning, clinicians have built trust with machine learning systems through experience.
Automatic learning or machine learning has the potential to improve clinical decision support systems. However, the impact of these tools depends on the doctors themselves applying and consulting them. Integrating machine learning tools into clinicians' workflows often presents challenges in clinical settings, especially those with time constraints and clinicians need to quickly evaluate recommendations.
The two main obstacles for the adoption of these models are: The difficulty that specialists have to generate confidence in machine learning models. And that many specialists see the lack of human experience with these systems and question their clinical value.
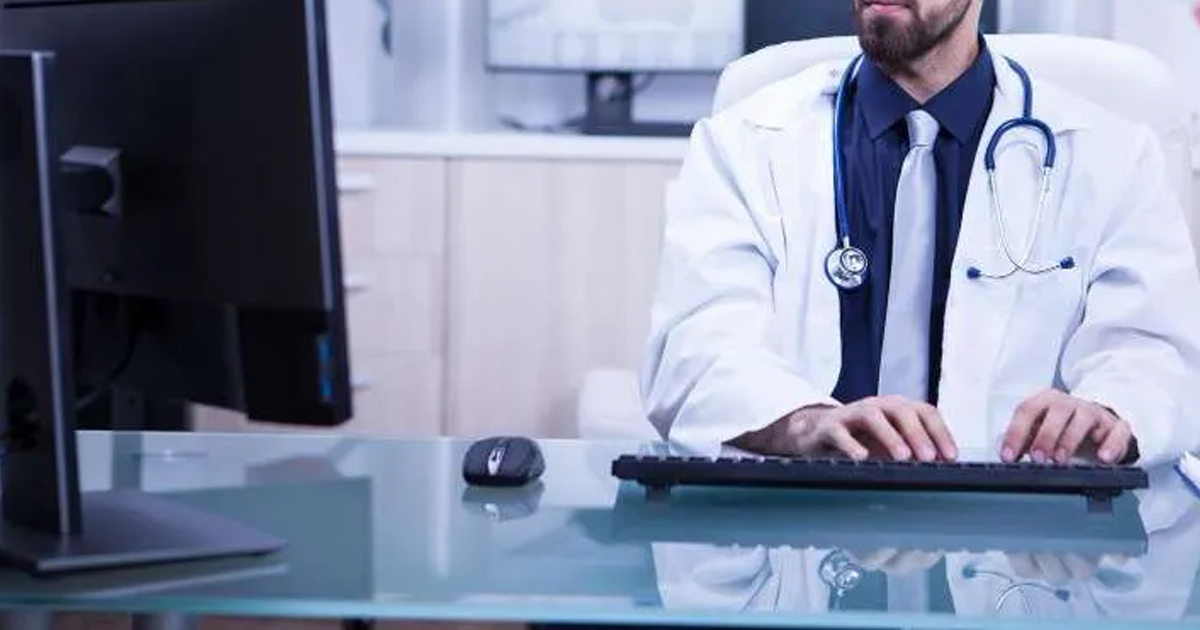
A qualitative analysis conducted by medical researchers from various universities and medical schools in the United States showed an analysis using coded interviews with physicians using a machine learning model for sepsis. The study sought to understand clinicians' perceived role of machine learning in acute clinical care and to understand the barriers to building trust with machine learning-based clinical recommendations.
Through interviews with medical and nursing staff, the study explored these situations in clinical contexts based on machine learning. One of the first findings of the study was that doctors recognized the improvements presented by machine learning systems with respect to other clinical support systems, however, they did not differentiate between conventional and machine learning-based systems.
The second finding was that “Clinicians perceived ML-based systems to play a supportive role both in diagnosis and beyond. Regardless of their understanding of the machine learning behind the system, clinicians generally responded to their alerts and integrated them into their diagnostic process. However, they saw themselves as holding ultimate responsibility for diagnostic and treatment decisions," the analysis explains.
The third theme identified was the mechanisms used by health professionals to build trust in the system, despite not fully understanding the models behind the system. “For doctors, I think just understanding [that] this is a machine learning tool and it does data mining, I think that will be more than enough,” explained one of the interviewed doctors.
The fourth theme was related to the enthusiasm of professionals with the potential of AI and machine learning-based systems to improve patient care, as well as the possibility of an excessive dependence on this class of automated systems.
Read more about this analysis in the following link: