Study published in npj Digital Medicine shows the estimation of age from sleep studies that use deep learning and manages to predict life expectancy.
Sleep disturbances increase with age and are predictors of mortality, according to the authors of the study. The gold standard diagnostic test for evaluating patient sleep is overnight polysomnography (PSG). This test is made up of physiological signals such as electroencephalogram, electrocardiogram, electrooculogram, chin and leg electromyogram, respiratory effort, and airflow. All of this data is recorded overnight while the patient sleeps and remains mostly immobile.
Although the main reasons for conducting clinical sleep studies are related to simple metrics such as sleep latency, proportion of time in sleep stages, sleep apnea, recent studies have shown deep learning methods to help efficiently and objectively to PSG analyses.
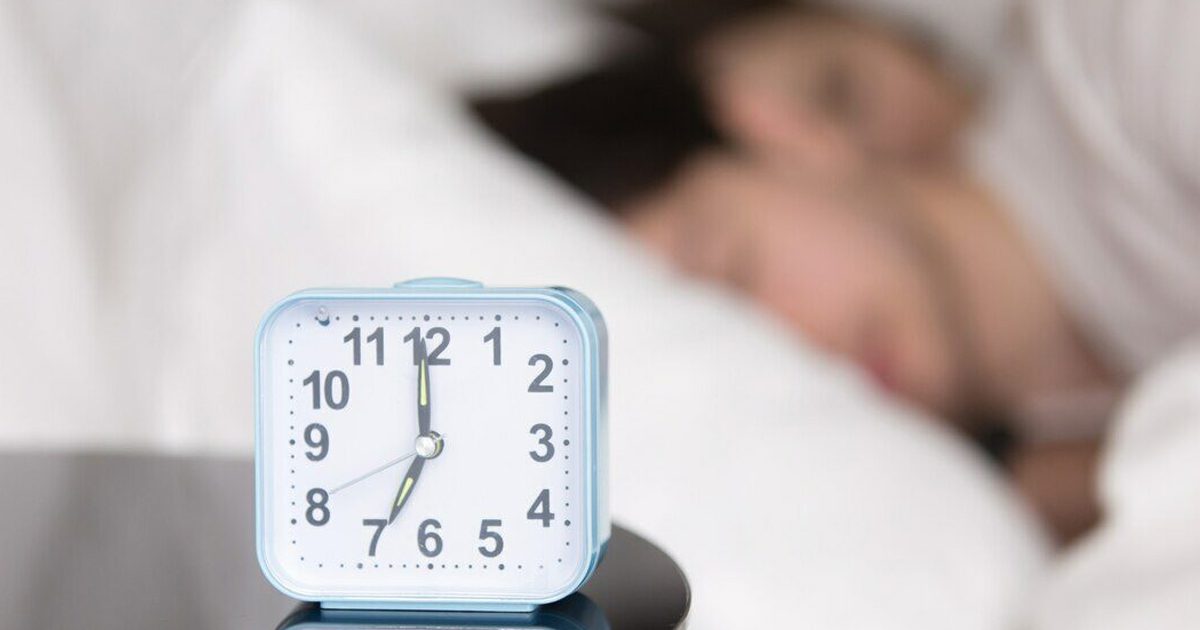
The new algorithms offer additional information such as higher resolution sleep stages and probabilistic measures. For example, taking into account that aging affects sleep architecture, it is possible to model age as an indicator of mortality risk using deep learning models.
The study presented by American and Danish authors shows deep neural networks that estimate age and mortality risk through PSG. They modeled 250 PSGs and tested them on 10,699 PSGs of men and women in seven different cohorts between the ages of 20 and 90. The estimation of ages had a mean absolute error of 5.8 ± 1.6 years and the basic measures of sleep scores had an error of 14.9 ± 6.29 years.
“A strength of this study is the inclusion of multiple cohorts, which is likely to increase the generalizability of our models. However, this is also a limitation, since measuring sleep with common instrumentation and in a more controlled environment could have better predictive power by reducing technical noise, first-night effect, variation in recording equipment , the location of the electrodes, the ambient temperature and external noise, etc.”, explains the study.
Learn more about this study: https://www.nature.com/articles/s41746-022-00630-9