The model aims to predict not only the complications and conditions that diabetes can cause in patients, but to predict the diagnosis of diabetes at least 3 years in advance.
Researchers developed a machine learning model that seeks to predict adverse outcomes related to complications with type 2 diabetes. They used health data from the Ontario health system in Canada to create the model. The decision tree on which the model is based was nurtured and trained with data from 1,029,366 patients, validated on more than 272,000 patients and tested on 265,406 patients,
The prediction of adverse effects in diabetic patients or those at risk of suffering from this condition, can strengthen health systems, by better managing emergency resources, hospitalizations and above all improving and carrying out strategies and comprehensive campaigns for prevention and promotion of better habits.
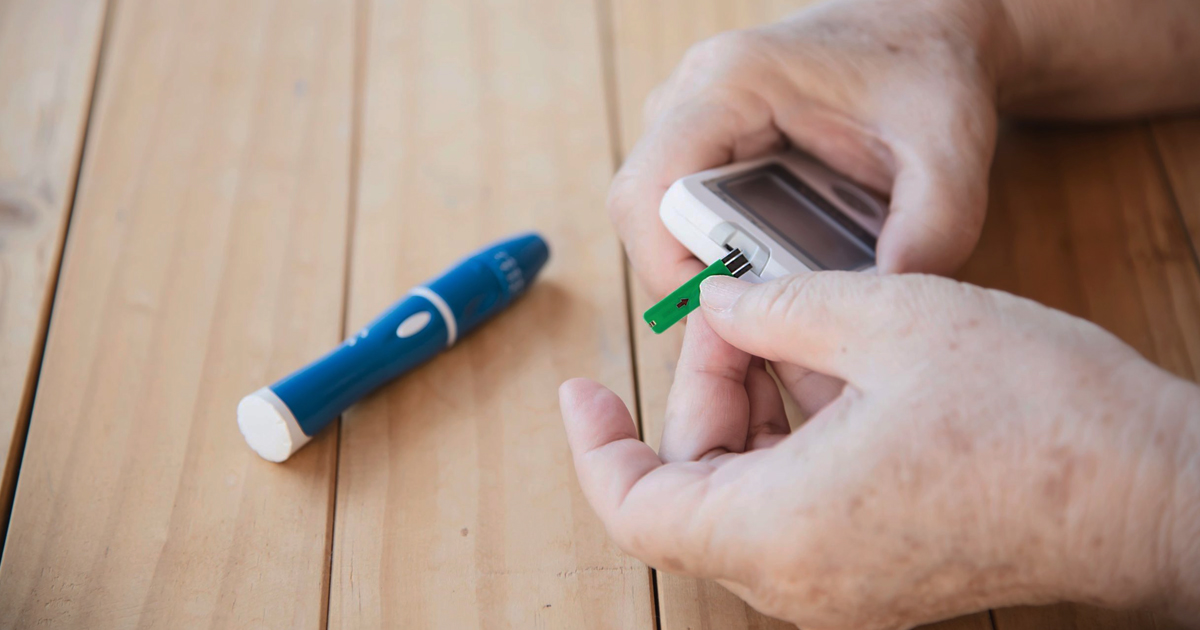
Controlling hyperglycemia and hypertension, as well as quitting smoking, are some of the ways to avoid complications in patients with diabetes. However, socioeconomic factors of patients, as well as social security models and universal health services, may prevent the prevention of complications on a massive scale.
The model developed predicts the risk of adverse outcomes at three years, through complications such as hyper- and hypoglycemia, tissue infection, retinopathy, amputations and cardiovascular events. “We aim to predict three years in advance, whether a patient diagnosed with diabetes will experience a healthcare visit from an adverse outcome due to a diabetes complication within a target three month prediction window. For this study, an adverse outcome is defined as at least one hospitalization or ambulatory usage associated with any diabetes complication during the target prediction window,” the authors explained in the article.
This prediction model was not performed with electronic clinical record data, but from administrative health data (AHD) sources, such as demographics, hospitalizations, physician visits, laboratory tests. “The final model has over 700 features from a variety of datasets such as demographics, census information, laboratory results, diagnosis history, physician billing claims, hospitalization and ambulatory usage, prescription medication history and others,” they explained.
“The proposed model was developed and tested on a large cohort of over 1.5 million patients with minimal exclusion criteria, capturing virtually all incidences of target adverse outcomes,” they further explained that the cohort is ethnically diverse to achieve broader representation from all regions of the world. The applicability of machine learning methods using AHD was thus demonstrated.
Read the full article here: https://www.nature.com/articles/s41746-021-00394-8