A machine learning method with a convolutional neural network (CNN) could work for the evaluation of small solid kidney masses in computed tomography (CT).
The article published in the American Journal of Roentgenology showed progress on this new diagnostic method. Researchers at Okayama University in Japan studied 1807 imaging sets of 168 solid renal masses less than or equal to 4 cm, diagnosed with CT in 159 patients.
The masses were classified as malignant (136), and benign (32), on a 5-point scale. Takashi Tanaka, lead author of the research explained the classification system: " "four were used for augmentation and supervised training (48,832 images), and one was used for testing (281 images)."
The CNN Model did not find a significant size difference between benign and malignant masses, however, the AUC value of the spinal corticomedullary was found to be greater than that of other phases. In addition, 88% accuracy was obtained in the images of that phase.
The results showed that the corticomedullary phase was an important predictor for determining whether the mass was malignant or not, compared to variables using other models such as the patient's age or mass size.
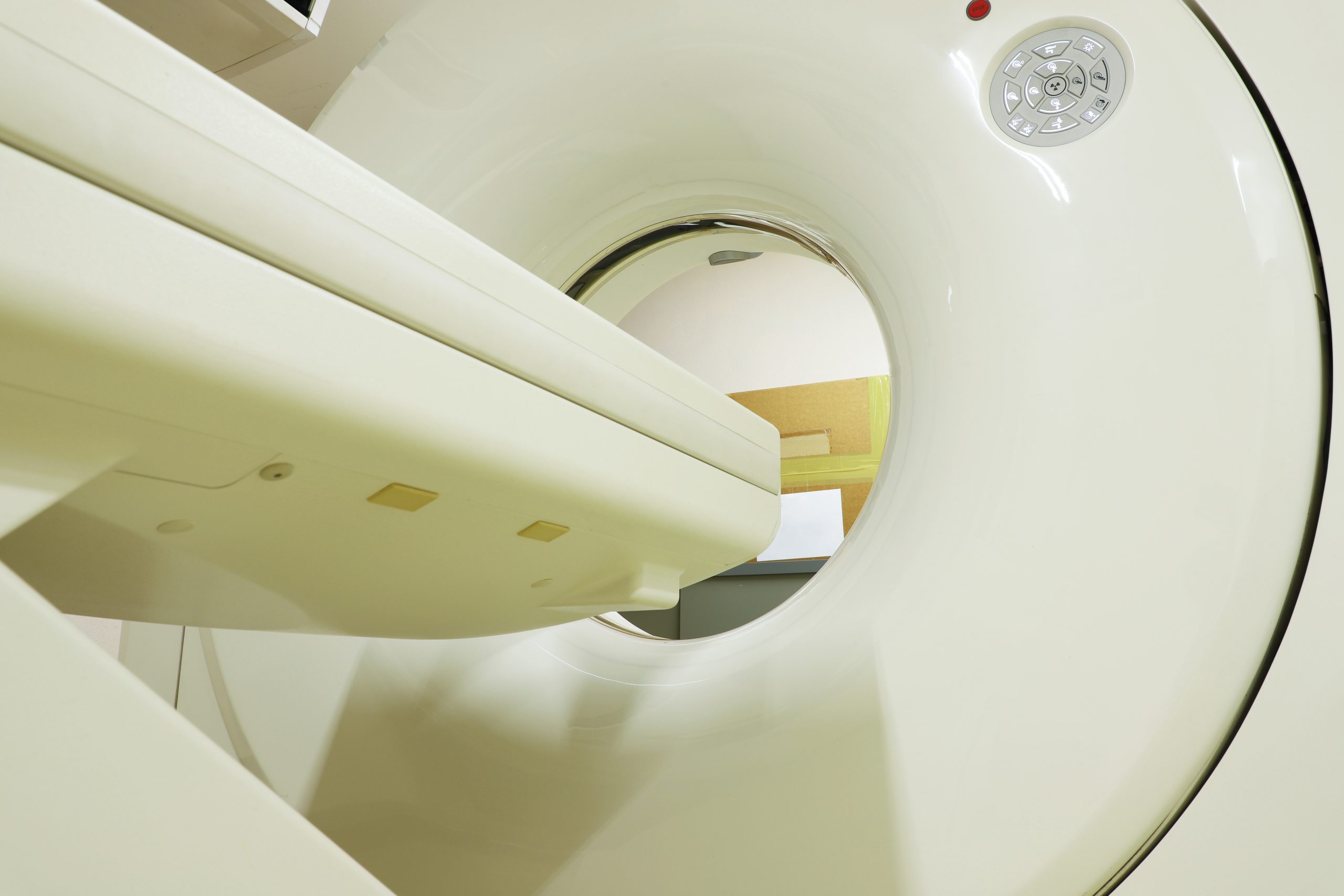