The scientific journal npj Digital Medicine, from Nature, published a guide to general concepts on the use of Artificial Intelligence (AI) in the medical sector, as well as definitions, functions and examples of its use.
Artificial Intelligence has gained great relevance over the past ten years. It is currently used in different industries, such as in the automotive or entertainment industry. Social media services use machine learning algorithms to function. In the field of medicine, the potential is greater, as it is possible to use it for drug development and design, for diagnostics, and for medical care, however, current evidence care has not been enough to further use.
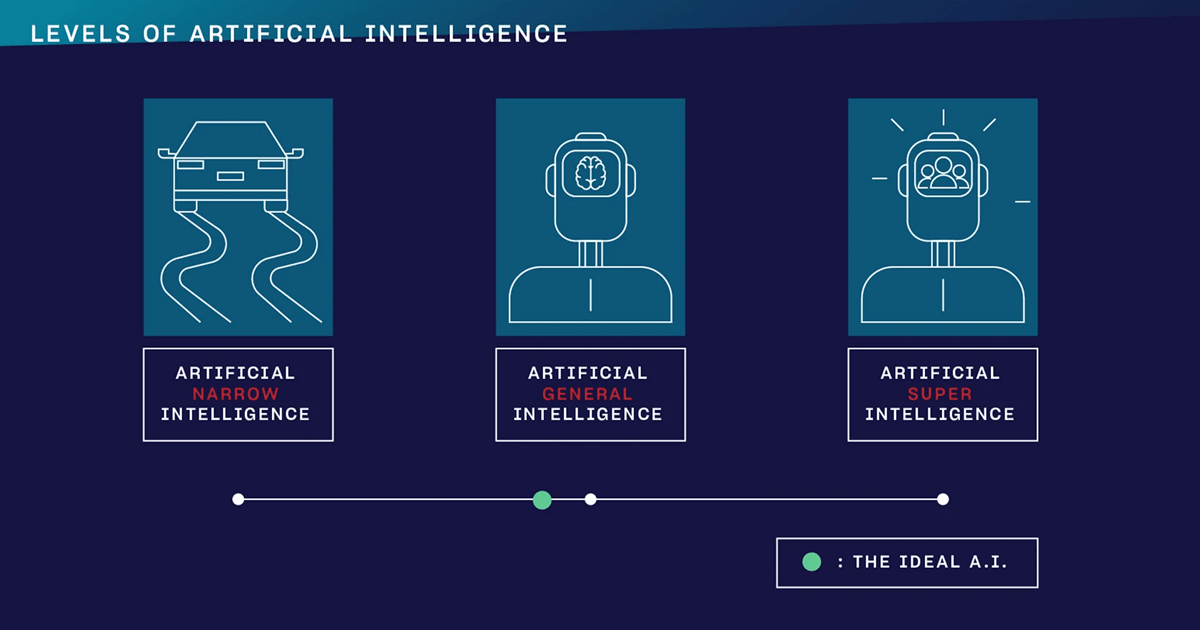
“There is no doubt that A.I. will have a beneficial role in healthcare and can penetrate the boundaries of adoption only if medical professionals serve as knowledgeable and supportive guides and leaders in the process,” the authors mention in the article.
To understand all the concepts involved in Artificial Intelligence, the authors developed a guide, which begins with the definition of AI, its levels and methods, as well as the differences between them. “AI is an interdisciplinary field spanning computer science, psychology, linguistics, and philosophy, among others.” The simplified definition is “machines that mimic the cognitive functions that humans associate with the human mind, such as learning and problem solving.”
Nick Bostrom, a philosopher at Oxford University, defined the three main levels of AI in his book Superintelligence: superintelligence:
- Artificial Narrow Intelligence (ANI): It is an algorithm that can develop a defined task with high precision. It is usually used to solve problems of sorting and grouping text, voice or images.
- Artificial General Intelligence (AGI): This type of intelligence could at some point have the same cognitive ability of a human being. Able to reason to discuss, memorize and solve problems.
- Artificial Superintelligence (ASI): This level does not exist and could theoretically have more developed capabilities than a human.
First, it is essential to know that AI works throughmachine learning, thanks to this technology, tasks that were previously complicated to perform within health care through traditional algorithms, now they are no more. This process involves providing the algorithm with a massive amount of data, and the algorithm through machine learning will create strategies to solve specific tasks.
There are also variations in machine learning:
- Supervised learning: It is used to define the task that the algorithm will learn based on data that we already know. For example, there are two sets of medical records of patients in a hospital, A and B. A contains a family history, laboratory or diagnostic results. B contains the same data, without diagnosis. So the ideal model to develop is one that learns to assign the right diagnoses to patients.
- Unsupervised learning: It is a built model that, to follow rules, however, the algorithm learns by itself, and is not modified. It is used, for example, to group tissue samples based on similar genetic values, or even for drug development.
- Reinforcement learning: This type of learning allows the algorithm to learn how to complete tasks without training. That is, the algorithm starts working alone, based on certain basic rules. An example cited by the authors is the following "the authors used this method to determine the dosage of the clinical trial, where the algorithm learned the appropriate dosing regimen to reduce the mean diameters of tumors in patients undergoing chemo and radiation therapy". This type of learning is directly applicable to health care.
The exponential growth of machine learning, both in real applications and in studies and research, was thanks to the rise of AI, when artificial neural networks began to develop within this field. From 2005 to 2014, 6.747 studies on machine and deep learning were published in Pubmed.com, however, just in 2019, 12,563 were published and in 2020, until May, 5.542 studies were published, showing the importance of both machine and deep learning and AI in academics and research.
Both concepts respond to different problems and apply to different situations. For example, machine learning can be used as a substitute for traditional statistical models, as it can include a large number of variables while traditional analyses were designed to enter data in smaller numbers. In prediction models, for example, in epidemiology, trained algorithms are used to create prediction models in infectious diseases.
In the article this difference is explained through an example of medical records and specific patient conditions: “let’s build a model that can cluster patients by diagnosis based on the data in their medical records. If a medical record contains the expression Type 1 Diabetes, a machine learning model will learn to put all such patients in the Type 1 Diabetes cluster. But a deep learning algorithm could learn with time without human input that patients with medical records that only mention T1D should also be assigned to the same group. Programmers of other machine learning algorithms should add these alternatives themselves”.
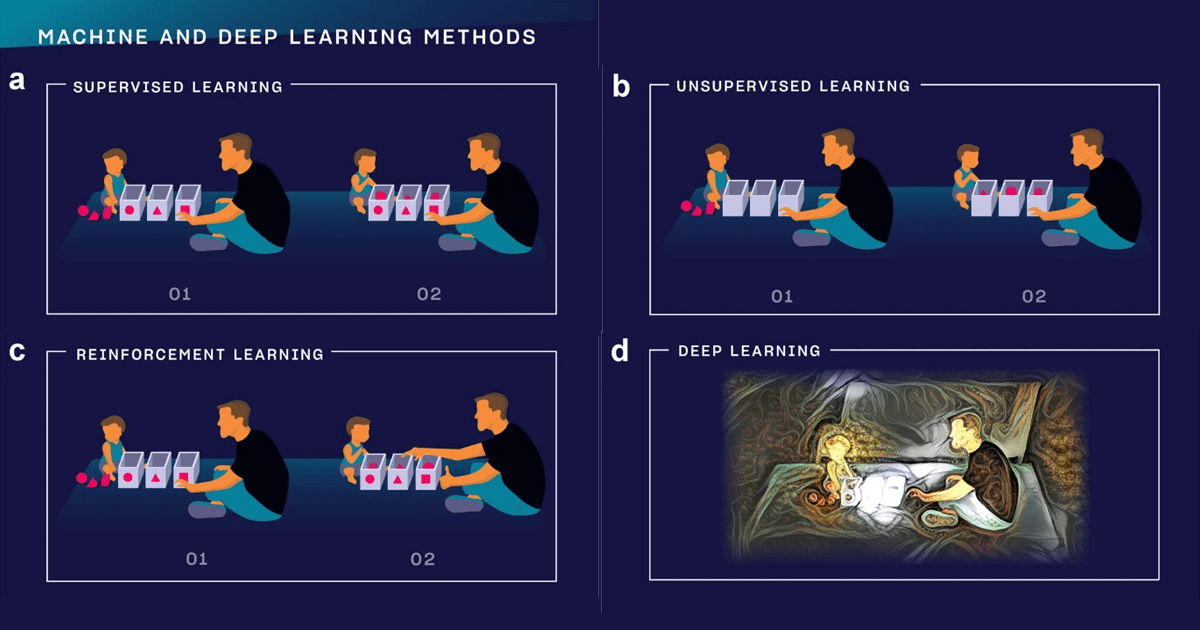
Both concepts respond to different problems and apply to different situations. For example, machine learning can be used as a substitute for traditional statistical models, as it can include a large number of variables while traditional analyses were designed to enter data in smaller numbers. In prediction models, for example, in epidemiology, trained algorithms are used to create prediction models in infectious diseases.