Massachusetts Institute of Technology (MIT) is developing a prediction model for early detection of breast cancer, based on Artificial Intelligence. It operates through pattern identification which allows to know the sickness predisposition with as much as 5 years in advance.
Researchers from the Computer Science and Artificial Intelligence Laboratory of the Massachusetts Institute of Technology have developed a prediction model for breast cancer based ondeep learning).
In this sense, the new functioning model will work over mammographies and the results they show in order to observe data which cannot be identified with the naked eye. The system compares data from the analysis so the changes in the breast tissue capable of being precursors to cancer can be more broadly studied, hence avoiding confusion with hormonal and biologic factors.
In order to establish the first pattern for analysis, more than 90,000 corresponding studies, made to 60,000 patients, were introduced, which generated the first knowledge base. With this kind of Artificial Intelligence, developed by Prof. Regina Barzilay, cancer could be detected up to 5 years in advance.
The real users of the solution, upload their information to help create their comparative history in which their results are evaluated according to the previous version and subsequently analyzed against the patterns previously defined in the knowledge base.
Studies can become very personalized due to the fact that, if the user presents any risk according of their record, complementary studies are requested for that particular user.
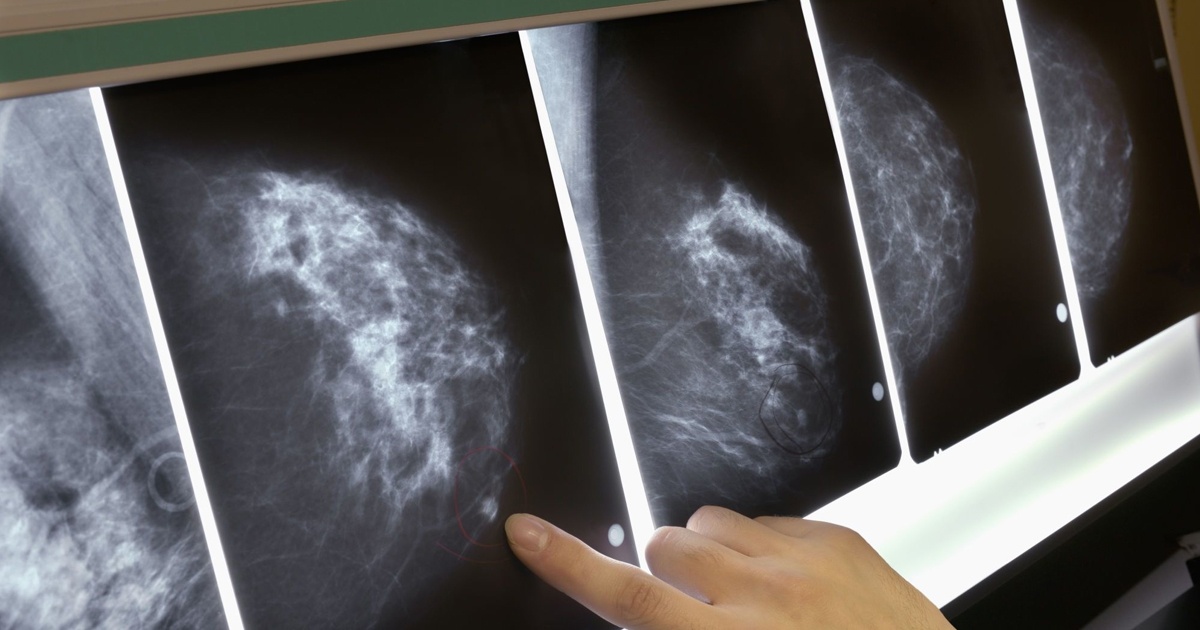
Being a digital platform, it eliminates racial differences between patients. According to a study published by the national Cancer Institute (NCI), black women are more propense to breast cancer than Caucasian women. The reason for premature deaths is the lack of attention given to the minorities and MIT aims to change that.
The aim of this project is to improve individual treatments and to become a helping tool to the specialist in the prevention and early treatment process. The implementation of this solution eliminates any bias generated during patients’ evaluation, which is focused on a specific part of the population and not equitably.
In order to achieve the objective of being a robust platform for infallible detection, it must start by creating a critical mass of measuring parameters and detected cases to which opportune attention can be provided and, with this, a better chance to keep alive by timely taking action.