An article published in Nature details a novel model for predicting blood glucose levels without the need for devices or needle sticks.
Type 2 diabetes is one of the biggest public health problems worldwide. Many treatments are aimed at reducing blood sugar levels, however, the most important thing is prevention and promoting healthy lifestyles, such as a good diet and physical exercise.
Research show that patients show greater motivation to improve decisions about their diet and exercise, when they have accurate and continuous data on their glucose levels. This is one of the main barriers, since continuous glucose monitoring is performed only through digital punctures, that is, an invasive technique.
Researchers from the Department of Biomedical Engineering at Duke University have developed a machine learning model for the prediction of interstitial glucose levels through non-invasive measurements. Through the glucose fluctuations reflected by the heart rate of the patients, they developed this model that combines 69 inputs for the prediction of glucose levels in the 16 study participants (patients with diagnosed prediabetes or with blood glucose levels normal to high).
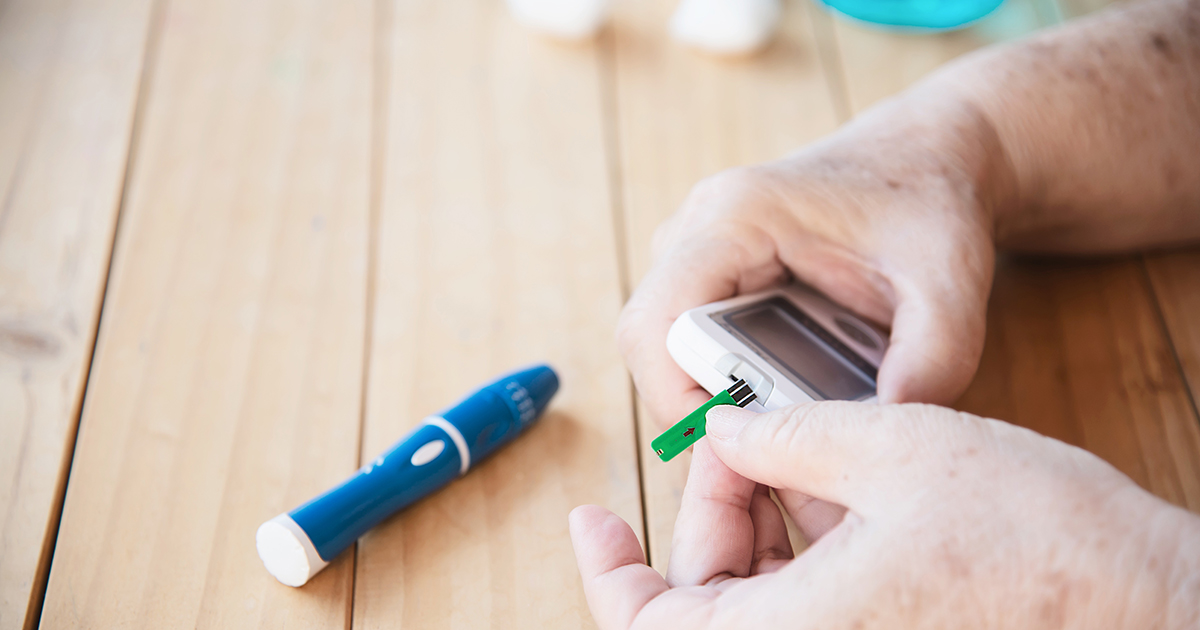
"Model inputs included demographic/historical data such as biological sex, food log (recorded by study participant), and last HgbA1c measurement, as well as biomarkers of stress, activity, and circadian rhythm," explain Leia Wedlund and Joseph Kvedar of Harvard Medical School in his editorial published in Nature.
The algorithm was trained using a continuous through monitor, which provided true interstitial glucose levels. The model used this data as a reference point to determine high glucose levels. Using data such as heart rate or daily diet, the model was able to predict the exact levels of interstitial glucose of the participants, this without using invasive techniques and registering an accuracy of 87%.
The original study “Engineering Digital Interstitial Glucose Biomarkers from Non-Invasive Smart Watches” by Brinnae Bent, et al. you can find it at the following link: https://www.nature.com/articles/s41746-021-00465-w