A recent study shows the evaluation of an Artificial Intelligence model capable of predicting different clinical risks in different hospitals in real time.
Recently the study “Prediction models based on machine learning for different clinical risks in different hospitals: Evaluation of in vivo performance”, was published in the Journal of Medical Internet (JMIR). Its main objective was to evaluate clinical risk prediction models in live workflows and thus be able to compare their performance in that environment with their performance when using retrospective data.
The importance of this study lies in the fact that they attempted a generalization of the results by applying the same research to three different use cases in three hospitals. Furthermore, the use of machine learning to develop clinical risk models is often limited to evaluations with retrospective data. This study shows the evaluation of the model through the use of data and clinical workflow in real time.
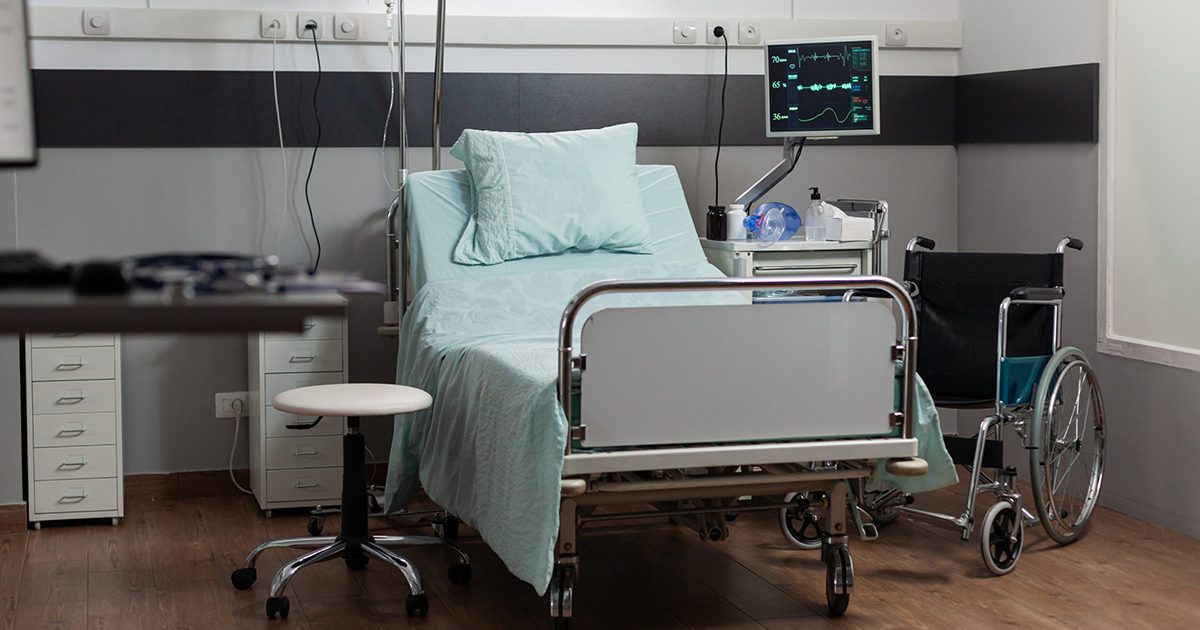
The prediction models used were trained for the prediction of clinical risk of delirium, sepsis and acute kidney injury, in three different hospitals and with retrospective data. Likewise, these models of machine learning models, specifically deep learning, were used to train a tool called transformer model.
“The models were trained using a calibration tool that is common to all hospitals and use cases. The models had a common design, but were calibrated using data specific to each hospital. The models were implanted in these three hospitals and used in daily clinical practice. The predictions made by these models were recorded and correlated with the diagnosis at discharge. Its performance was compared with evaluations on retrospective data and interhospital evaluations were carried out, ”explains the study.
The results showed that the performance of the models using clinical workflow data was similar to using retrospective data. The average value of the Receiver Operating Characteristic Curve - ROC or receiver operating characteristic curve (AUROC), had a decrease value of 0.6% from 94.8 to 94.2%.
“Cross-hospital assessments showed very poor performance: the mean AUROC decreased by 8 percentage points (from 94.2% to 86.3% at discharge), indicating the importance of model calibration with deployment hospital data” , shows the study.
Thus, the authors concluded that calibrating the model with data from the various hospitals achieves better results and model performance in live workflows. Check out the full study at the following link: