Researchers from the Center for Mathematical Modeling (CMM) of the University of Chile, created an Artificial Intelligence (AI) model, capable of optimizing the management of the hospital agenda.
Requesting and scheduling appointments for medical consultations wastes time and resources in hospitals and medical offices when patients do not show up. Faced with this problem, researchers from the CMM of the University of Chile have developed an AI-based system, which aims to increase efficiency in hospitals. They have even tested it for three months in three hospitals in the country.
The researchers received financial support for the development of the research, through a promotion for scientific development. Jocelyn Dunstan and Héctor Ramírez were in charge of creating software designed to increase and improve efficiency in hospitals.
Thanks to machine learning technology, the software seeks to know the probability that patients will not show up at the time of their appointment, this thanks to the data entered on the assistance history of each patient, geographic factors such as the distances between homes and hospital, and other social factors.
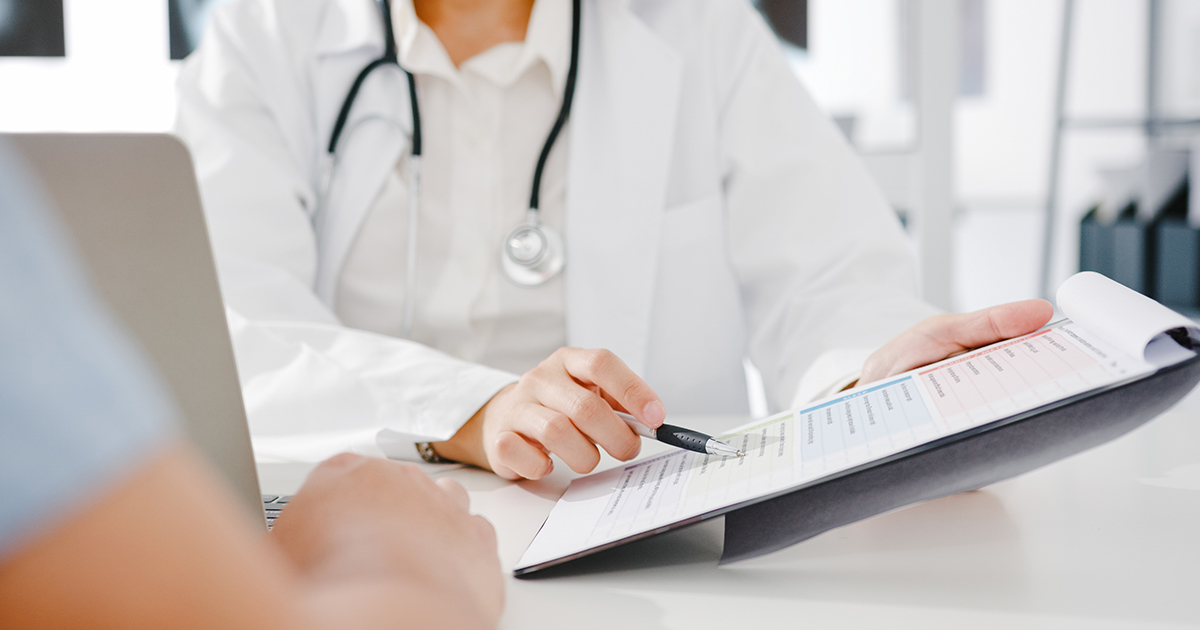
“We are concerned with making this model try to call as little as possible, but with the highest possible attendance confirmation effectiveness,” explains Ramírez. Dunstan explained that they require a large amount of historical information from patient data to be able to train these types of models and understand the pattern or profile of the person.
The model was applied in three hospitals: the Luis Calvo Mackenn Pediatric Hospital, the Talca Regional Hospital and the Cordillera Oriente Health Reference Center. The work consisted of intervening in the medical agenda of the hospitals with the intention of testing the predictive model in a real way. This exercise helped the models get more data, improving their efficiency.
The scientists explained that the main challenge in the processes of automatic learning and the entrainment of predictive models consists of heterogeneity, since each hospital works differently and its patients have different profiles. “For this reason, we decided not to make the same model for all hospitals, but to differentiate it by establishment, with its own data. Apart from that the specialties also have different behavior of patients”, explained Ramírez.
It also depends on each patient, the way in which they are alerted about their next visit to the doctor, some prefer it by phone, through WhatsApp, or SMS. And in the same way it depends on each medical specialty. There are differences in the behavior of patients in appointments with an ophthalmologist or a gynecologist, for example.
In one of the hospitals, the pilot test of the project managed to reduce the insistence of patients who are reminded of their appointments through telephone calls from 20.3% to 12.5. "The natural thing for us is that for the future this would be something used by most public hospitals, and in some private institutions, where efficiency when contacting patients is very relevant," Ramírez concluded.