The application of a model developed by Google to detect tuberculosis with the same precision as radiologists was explored in a study published by the Radiological Society of North America (RSNA).
In the study, deep learning, or deep learning, was used for the detection of active pulmonary tuberculosis on chest radiographs, which was consistent with the clinical performance of radiologists. For this, the model was trained using chest radiographs from 1996 to 2020 from 10 countries. In this way the model of deep learning was jointly evaluated through trials in four countries, China, India, the United States, and Zambia.
The World Health Organization recommends chest radiographs to facilitate the detection of tuberculosis, however, the interpretation of this type of radiograph continues to be very limited in various regions of the world. Hence the importance of the study, since according to the WHO, tuberculosis affects 10 million people each year, and 1.5 million people die for reasons related to tuberculosis annually, despite the fact that it is preventable and, above all, curable.
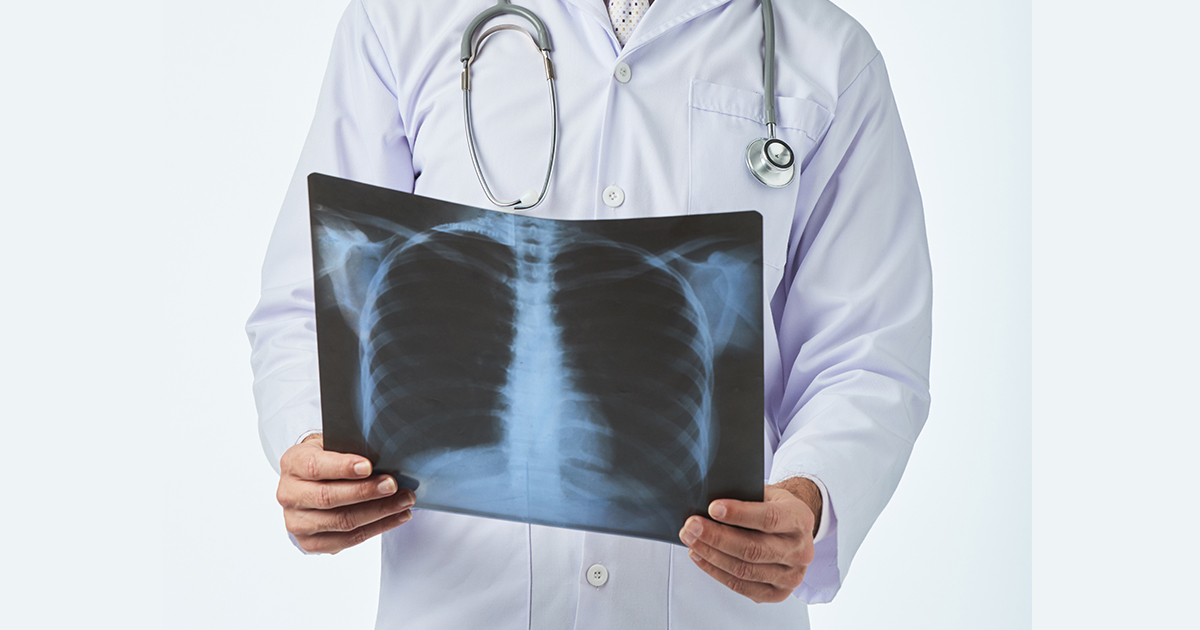
The objective of the study was to achieve sensitivity targets for 90% or specificity for 70%, to meet the guidelines required by the WHO for these models. After various tests, the model managed to measure results on par with the 14 participating radiologists, achieving a sensitivity of 88% compared to 75 of the radiologists, and a specificity of 70% by 84 of the radiologists.
Also, the model was tested with a mining population from South Africa, because this population has a high prevalence of pulmonary abnormalities and chronic obstructive disease. Similarly, the model remained comparable to the work of radiologists.
“Our study has limitations. First, this study was retrospective; prospective validation studies are being planned to better understand the challenges of integration in real-world workflows. As all of our data sets had a relatively high prevalence, we will need to assess their performance in populations with a lower prevalence,” the authors explain.
Likewise, the study details that the performance of the model is not affected by changes in prevalence, changes in prevalence are associated with the severity of the disease, which may affect the sensitivity or specificity of the model.
Finally, the cost analysis is a simulation that makes simplified assumptions, such as that DLS performance is not affected by changes in prevalence. In practice, changes in prevalence may be associated with disease severity, which may affect the sensitivity or specificity of the DLS.
Check the details of the study in the following link: