Mayo Clinic researchers developed a machine learning model to differentiate patients with acute cholangitis and hepatitis associated with alcoholism.
A study presented by the Mayo Clinic showed how a machine learning model can support medical decision-making by helping specialists differentiate between alcohol-related hepatitis (AH) and acute cholangitis (AC) using simple laboratory variables.
Despite the differences of both conditions, the clinical similarities can complicate the work of doctors due to the symptoms presented. The study included 459 adult participants, who attended the Mayo Clinic, Rochester, of whom 265 had AH and 194 AC.
"This study was motivated by seeing many medical providers in the emergency department or ICU struggle to distinguish between acute cholangitis and alcohol-associated hepatitis, which are very different conditions that can present in similar ways," explained Joseph Ahn, a gastroenterologist at Mayo Clinic, Rochester.
The analysis took into account ten laboratory variables: white blood cell count, hemoglobin, mean corpuscular volume, platelet count, aspartate aminotransferase, alanine aminotransferase, alkaline phosphatase, total bilirubin, direct bilirubin, and albumin. As well as eight machine learning models such as decision tree, naive Bayes, logistic regression, artificial neural networks, among others.
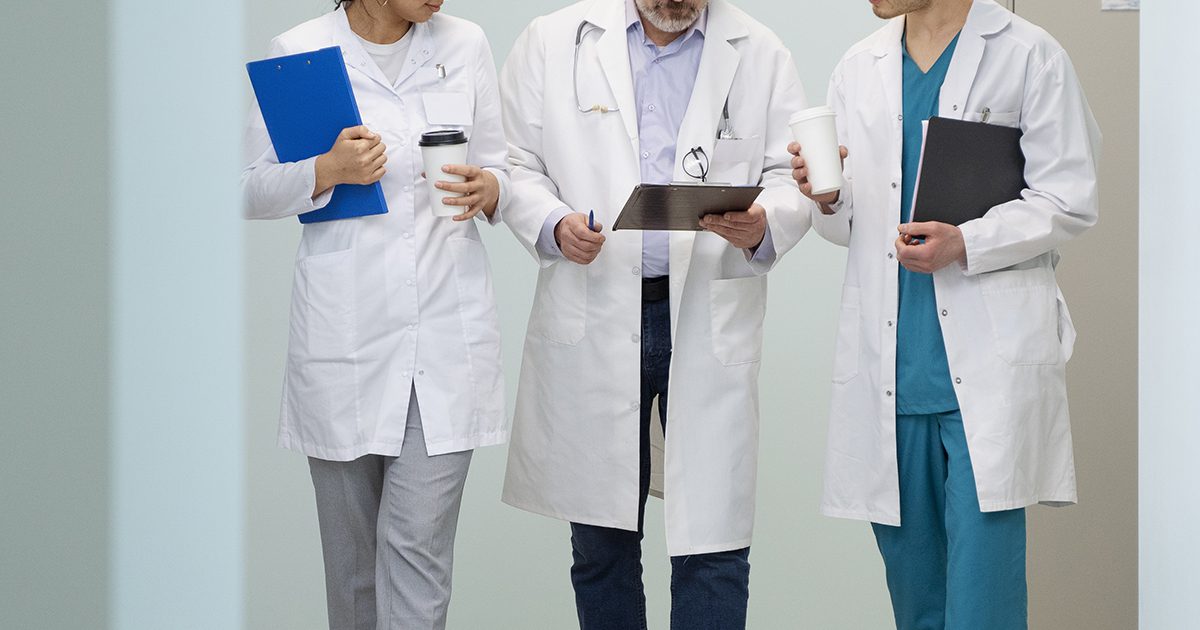
"We developed and trained machine learning algorithms to distinguish the two conditions using some of the routinely available lab values that all these patients should have," explained Dr. Ahn.
In this way, the models were trained with these laboratory variables. Machine learning models demonstrated accurate returns with 0.923 and area on the curve above 0.986. That is, the validation obtained a precision 93%. It also complied with external validations and was accurate using another patient database, however, the accuracy percentage decreased by three points.
The study highlighted the use and clinical potential of using trained machine learning algorithms. In this way, in addition to achieving greater precision for the diagnosis of these conditions, patients would not have to undergo invasive procedures, which can delay diagnosis.
Check out the full article here: https://secure.jbs.elsevierhealth.com/pb-assets/Health%20Advance/journals/jmcp/JMCP3718_proof.pdf