The Artificial Intelligence (AI) model uses electrocardiogram images to diagnose different heart diseases.
Researchers at the Yale Cardiovascular Data Science (CarDS) Lab at Yale School of Medicine have developed an AI-based model that helps improve the diagnosis of cardiac arrhythmia through the analysis of electrocardiogram (ECG) images.
The team led by specialist Rohan Khera, assistant professor of cardiovascular medicine, explained that most AI-based tools are designed for individual clinical disorders, so they have limited utility.
In this case, the solution designed by CarDS Lab aims to improve ECG interpretation even remotely. The results of the research were published in Nature: https://www.nature.com/articles/s41467-022-29153-3.
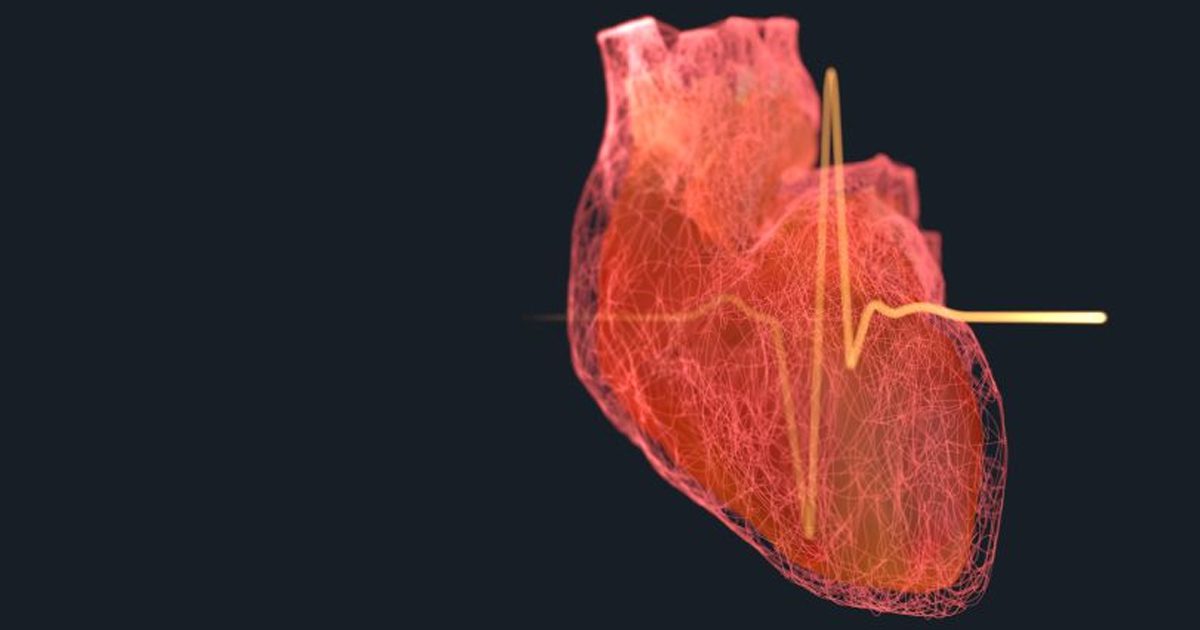
The research details that it is possible to improve care and diagnosis based on ECG in remote environments, for this reason they developed a multi-brand automated diagnosis model for electrocardiographic images, more suitable for a broader use”.
The study shows that, through 2,228,236 12-lead ECG signals in 811 municipalities in Brazil, they are transformed into ECG images in different lead conformations to train a convolutional neural network (CNN).
The data correspond to patients in Brazil who were treated between 2010 and 2017, of which one in six patients was diagnosed with heart rhythm disturbances.
“Current AI tools rely on raw EKG signals rather than stored images, which are much more common since ECGs are often printed and scanned as images. Furthermore, many AI-based diagnostic tools are designed for individual clinical disorders and thus may have limited utility in a clinical setting where multiple ECG abnormalities coexist,” Khera explained.
Another point that Khera highlighted was that it is an intelligent model that does not depend on specific ECG designs, since it has the ability to adapt to new designs. This way you can enhance and support the work of expert human readers.
In this way, the model has the potential to expand the application of AI in clinical care, specifically in ECG-based techniques, according to Veer Sangha, lead author of the study.