The study aims to understand, predict and prevent suicidal behavior risk through structured and unstructured data in electronic medical records (EHR) and specialist notes, respectively.
Researchers from psychiatry departments at Boston institutions such as Harvard University, Boston Children's Hospital, and Massachusetts General Hospital conducted a study on predicting suicide risk through structured and unstructured patient data.
The study published in npj Digital Medicine journal shows the development of a clinical risk prediction model, developed through structured information from ECE and unstructured information such as doctors' notes, which was interpreted through natural language processing (NLP).
In this sense, structured information includes information such as diagnoses and medication, so it is important to add unstructured data such as medical notes, so that the predictive model understands the value of each data classification and the interactions between the two.
The study titled: Structured-Unstructured Predictive Interactions in EHR Models: A Case Study of Suicide Prediction, contemplated three objectives:
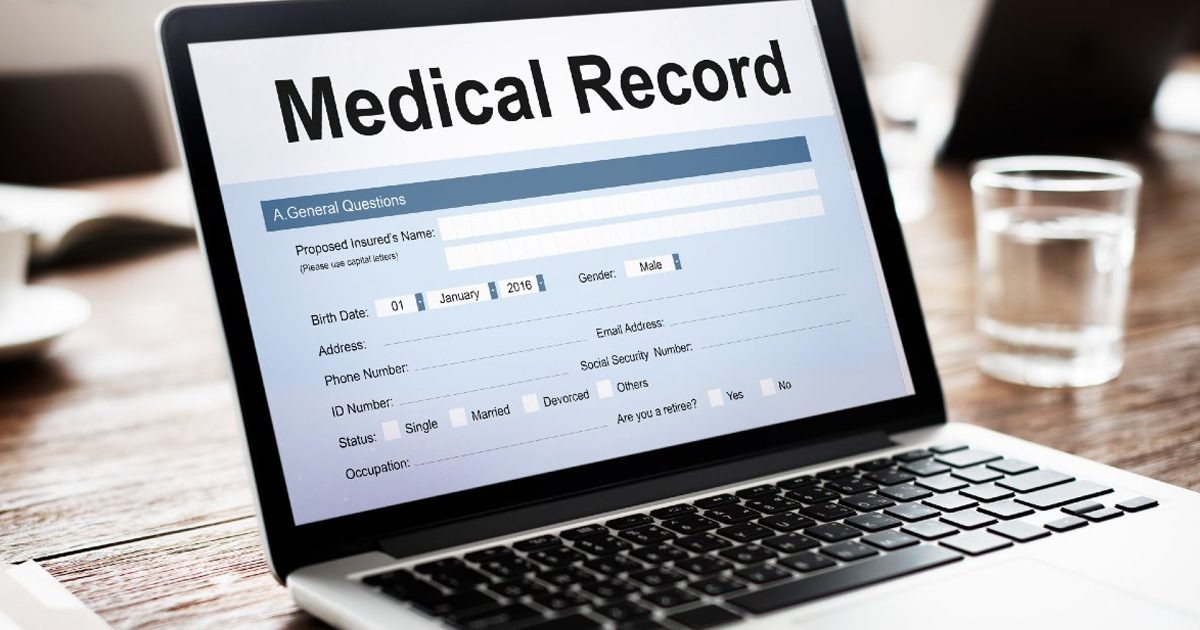
- To compare the predictive value of structured and unstructured ECE data as independent data sets for predicting suicide risk.
- Evaluate the increase in prediction performance when integrating structured and unstructured data using several models: Naive Bayes Classifier (NBC) and Random Forest (RF).
- Identify pairs of structured and unstructured characteristics in which the interaction between the two characteristics differs substantially between populations with suicide attempts and people without attempts.
The application of the inclusion and exclusion criteria produced 1 million 625 thousand 350 training subjects for the 99% models, corresponding to non-cases and 16 thousand to cases, that is, the 1%.
The most commonly found captured variables in the dataset were impulse control disorder, bipolar disorder, schizoaffective disorder, and opioid dependence or abuse.
In this way it was possible to identify structured and unstructured data variables on patients at risk of suicide or suicidal behaviour. Based on these data, it is possible to develop effective suicide prevention strategies.
Check the full study at the following link:
https://www.nature.com/articles/s41746-022-00558-0