Risk scores are used to make more informed clinical decisions, and machine learning implementation is helpful in identifying important predictors for scoring.
With risk scores, clinicians can make faster assessments of a patient's risk of achieving scores associated with key predictors of risk. On the other hand, machine learning improves these processes, by having tools with greater capacity for the selection of variables. A study published in PLOS Digital Health, made a connection between machine learning tools (AutoScore) and risk scoring tools (ShapleyVIC).
For the study, the authors proposed a variable selection mechanism since connecting both tools directly could affect interpretability and predictive performance. The developed model is tailored to risk scores and was integrated into an automated framework for risk score development.
The study demonstrated how the proposed method can help researchers understand the 41 candidate variables for predicting outcomes. "We have presented a useful tool to support transparent high-risk decision-making," the study explains.
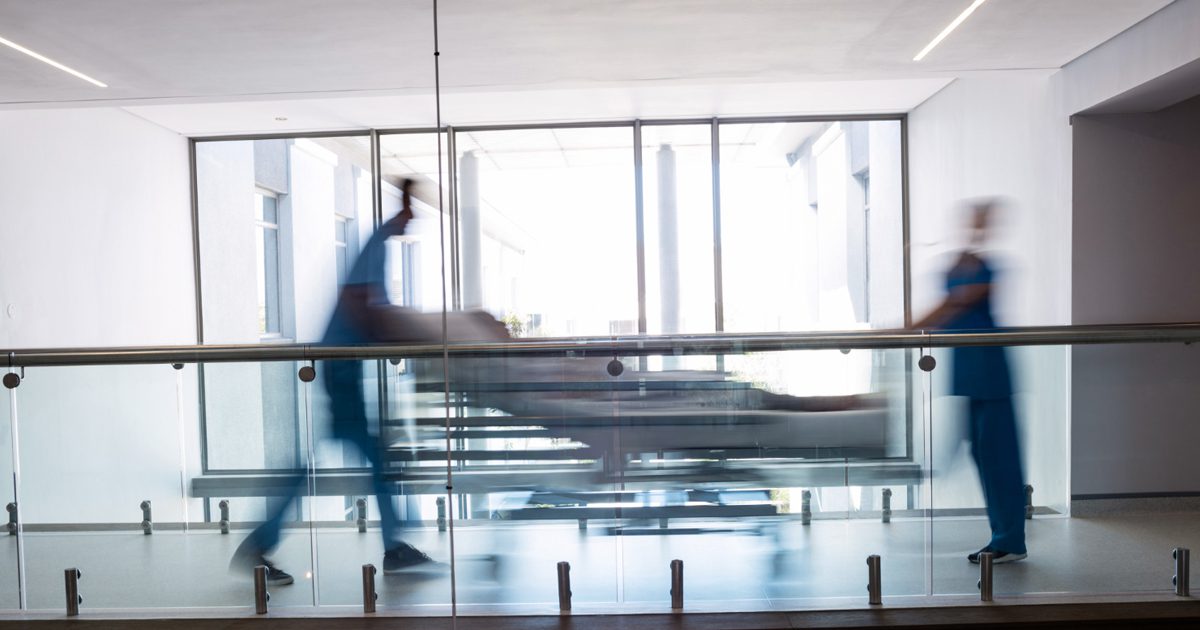
This investigation focused on premature death or unplanned readmission after hospital discharge. The variable selection method obtained 6 variables from 41 candidates to develop the risk score of an acceptable performance, this had a performance similar to that of a 16-variable model based on machine learning.
“Our work contributes to the recent emphasis on the interpretability of prediction models for high-risk decision making, providing a disciplined solution for the detailed assessment of variable importance and the transparent development of parsimonious clinical risk scores,” explains Dr. Article.
One of the models used for the study, AutoScore, has been tested in various settings such as to derive risk scores in emergency department triage, as well as to provide scores on survival after out-of-hospital cardiac arrest.
See more details about both models and their results by reading the full study at the following link:
https://journals.plos.org/digitalhealth/article?id=10.1371/journal.pdig.0000062