Precision medicine combines various technologies to guide healthcare professionals to make better clinical decisions.
Omics technologies arose from research on the human genome, and are used for processing complex samples and generating large amounts of data for medical research. These types of technologies are associated with precision medicine and molecular signatures.
The article: “Cross-Platform Unique Prediction Procedure: A Statistical Machine Learning Framework for Wider Implementation of Precision Medicine”, published in npj Digital Health, explores new approaches to using omics data to independently predict patient outcomes.
The study presents the Cross-Platform Omics Prediction (CPOP), which is a regression model that is able to use omics data to predict patient data independently of the platform and over time. The publication details that CPOP improves the traditional prediction framework by using gene-based features.
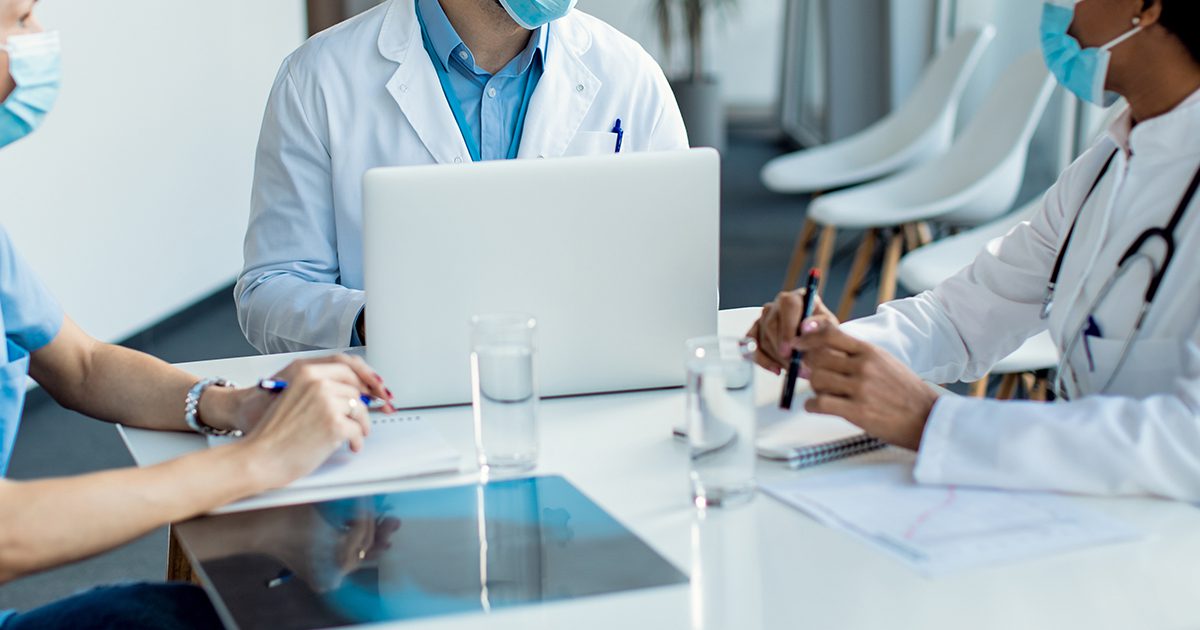
In this way, the CPOP was developed as follows, taking into account three specific phases. First, the construction of features based on proportions, this means that they can be used on various platforms as their name indicates. The second step is that the selection process incorporates functions that measure the stability of functions in multiple data sets. And finally the third step is to select features that have estimated effects on multiple data sets.
In this sense, the model was built for the analysis of stage III melanoma and used a newly generated data set based on an independent cohort. The most relevant aspect of this research is that the authors developed an interactive web portal where the loading of data is illustrated, and the obtaining of risk predictions in patients with stage III melanoma: http://shiny.maths.usyd.edu.au/CPOP/.
The molecular risk calculator allows gene expression data to be uploaded to assess the prognosis of stage III melanoma patients.
The work was carried out by the Melanoma Center of Research Excellence, Melanoma Research Institute Australia and the University of Sydney. Learn more about this methodology in the full article: https://www.nature.com/articles/s41746-022-00618-5