A recent study by the Indiana University Regenstrief Institute showed the application of machine learning models to predict the probability of hospitalization for COVID-19 in infected patients.
Machine learning has been an important medical resource to support decision-making during the pandemic in the United States. Researchers at the Regenstrief Institute published a study detailing how machine learning models, trained on clinical data from across the state of Indiana, were able to predict the likelihood of hospitalization for people who contracted COVID-19.
"What we thought to do was take advantage of this urgent need to create machine learning solutions that could help health care decision makers make general decisions about the level of health of the population they serve," explained Dr. Suranga Kasturi, first author of the study.
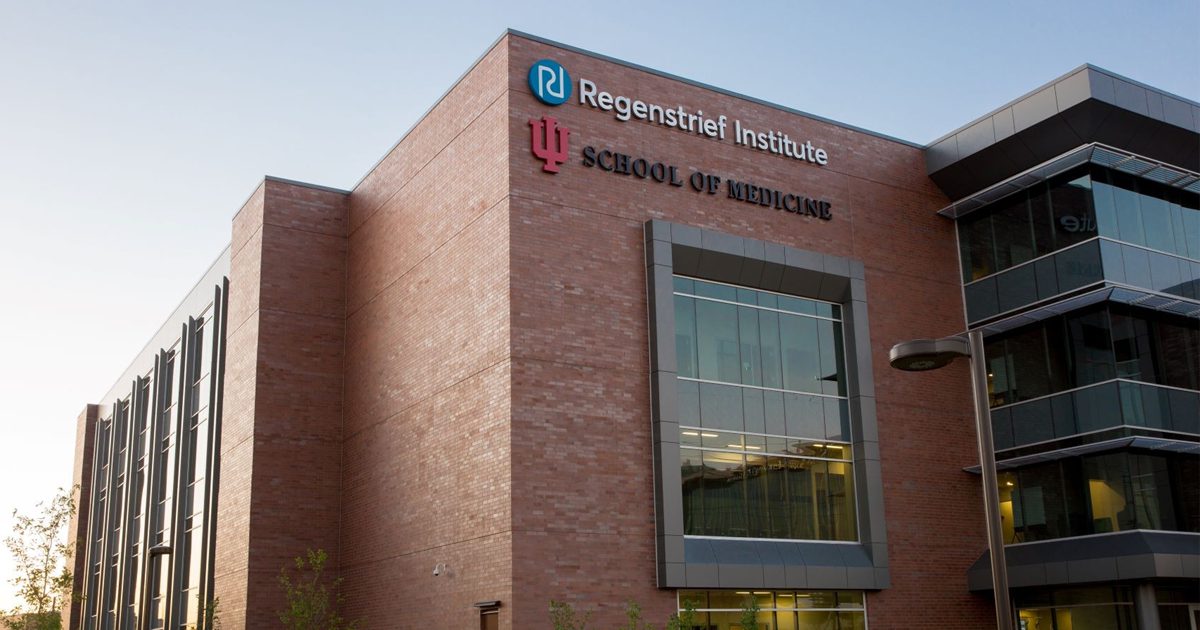
The objective of the study was to develop technology that favors the effective use of available resources. "Whether it's the state, or the county, or some other region, you can effectively estimate for the next week or six weeks how many health care resources you're going to need," Kasturi said.
The clinical data used for the study are part of the Indiana Network for Patient Care (INPC), which contains more than 14 billion pieces of clinical patient information. For the study they collected information from 96 thousand 26 patients.
“In the context of this study, having machine learning solutions help us harness vast amounts of data to help inform broad decisions that affect population and public health. Things that we just couldn't do if there wasn't an AI-powered system to work with such large amounts of data."
In this way, the developed models achieved a great performance in their predictions, however, when specifying the variables in subpopulations of the state, there were some disparities. As explained by Dr. Kasturi, the models worked well, however, there is a lot of room for improvement to enhance the performance of the algorithms.
“The next step would be to address those disparities, ensuring that the model provides fair and equitable care. Once that happens, we'll be able to have broader conversations in terms of how it can be integrated into existing public health workflows."
The study is available at the following link: https://www.jmir.org/2021/11/e31337