A team from the University of Ottawa (uOttawa) has created a model enabled by Artificial Intelligence (AI) to diagnose birth defects on obstetric ultrasound images.
A study conducted by a team from the uOttawa School of Medicine shows the use of a deep learning model as an assistive tool for fast and accurate reading of fetal ultrasounds. The study was published in the scientific journal PLOS One: https://journals.plos.org/plosone/article?id=10.1371/journal.pone.0269323.
The objective of the study was to demonstrate the potential of deep learning for the early and reliable identification of cystic hygroma, through ultrasound performed during the first trimester. This condition causes the embryos to suffer changes in their lymphatic vascular system and does not develop properly. In this sense, it is a rare and life-threatening disorder, which requires early diagnosis.
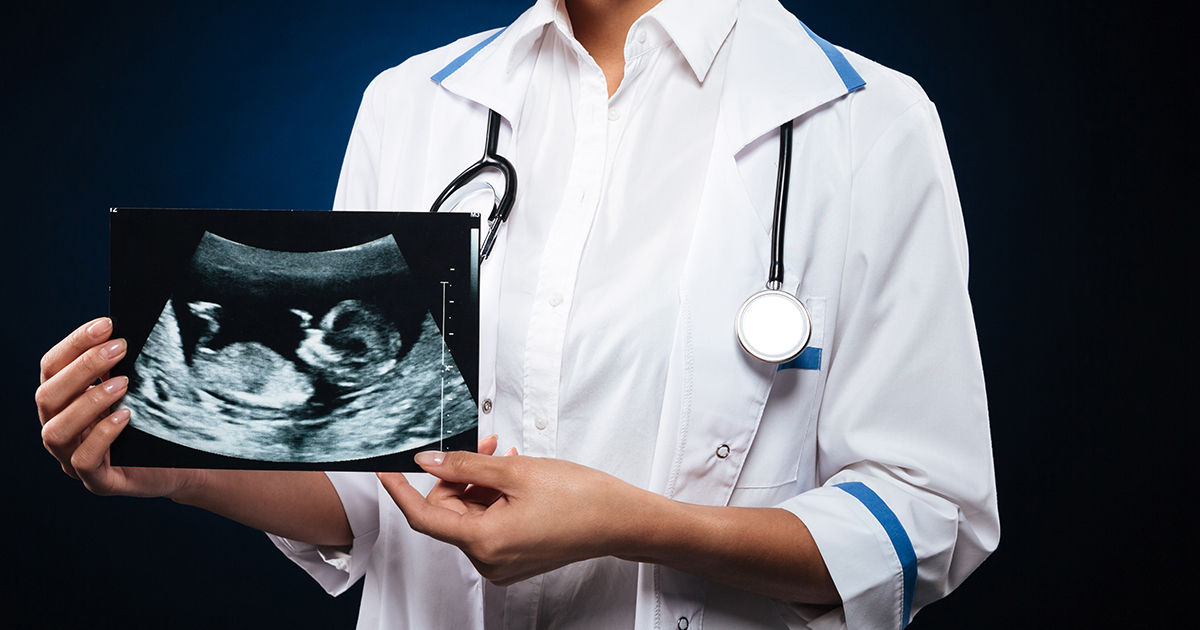
This defect is easy to spot at a routine ultrasound appointment, however the researchers sought to test how effective AI was at pattern recognition in these types of images. For this they used around 300 fetal ultrasounds obtained from the Ottawa Hospital.
Subsequently, the images were analyzed using the DenseNet model, which was able to correctly identify the cases of cystic hygroma. Heat maps were also generated to evaluate the interpretability of the model. Finally, the model achieved an overall accuracy of the 93%.
“The model was outstanding, even with a small number of training images. So potentially what we showed was that in the field of ultrasound we can use the same tools for image classification and identification with high sensitivity and specificity,” said Dr. Mark Walker, lead of the study.
Dr. Walker explained that the team now aims to develop a cloud-based database for uploading obstetric ultrasound images to help specialists in low- and middle-income countries easily interpret these types of diagnoses.