A study showed that through medical images of the body silhouette it is possible to measure the distribution of body fat and the risk of suffering from cardiovascular diseases.
The distribution of fat in the body is clinically important however it is not always routinely assessed in practice. For example, the body mass index (BMI), is an indicator of the total fat load, which is used to define obesity in clinical practice and as an evaluator of the risk of cardiovascular diseases and type 2 diabetes. Although it is than a useful indicator, fat distribution may be more helpful in defining metabolic risk profiles.
MIT researchers developed a deep learning model that uses magnetic resonance imaging (MRI) to identify fat deposits in the body to better identify metabolic risk. Previous studies had used images such as: computed tomography (CT) and dual energy X-ray absorptiometry (DEXA).
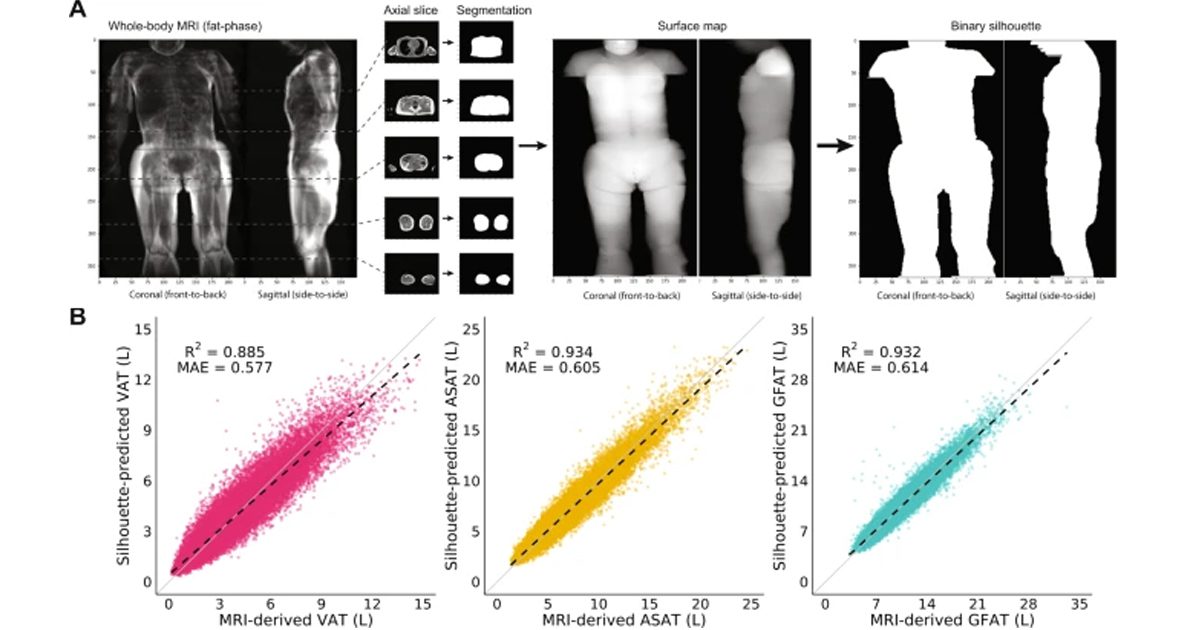
The study involved 40,032 people from the UK Biobank's sub-study of body MRI. The mean age of the participants was 65 years, and 20,597 (51%) were women. For the study, they took into account the following variables in the recovered images: visceral adipose tissue (VAT), which is the fat that surrounds the internal organs; abdominal subcutaneous adipose tissue (ASAT), that is, the accumulation of fat just under the skin; and gluteofemoral adipose tissue (GFAT).
For the study, coronal and sagittal silhouettes of each patient were generated, performing segmentation of the body contour in axial MRI. In turn, they calculated a surface map of the resulting segmentation volume. Finally, three-dimensional surface images were projected into two-dimensional images and pixel intensity converted to binary values.
Finally, the resulting silhouettes were used as inputs for training a convolutional neural network, capable of predicting VAT, ASAT and GFAT volumes using MRI measurements and a cross-validation procedure.
"Cross-validated deep learning models trained on these images, using previously computed whole-body MRI estimated volumes as truth labels, demonstrate highly accurate estimation of VAT, ASAT, and GFAT volumes," the authors explained.
Check the full study at the following link: