A study on the use of machine learning, via the electronic medical record (EMR) of patients with cardiovascular conditions, was published in Nature.
The EMR contains information about patients' physiological aspects and information. When the physiological level reaches a critical point that requires examination, there are beliefs of the specialist medical staff that serve in decision making.
Two types of data are obtained through machine learning: first, those related to specific actions of the medical practice, expressions in decision-making, diagnoses, referrals, consultations or non-routine procedures; then those data "not initiated by the physician", which include physiological orders and intakes of the patient.
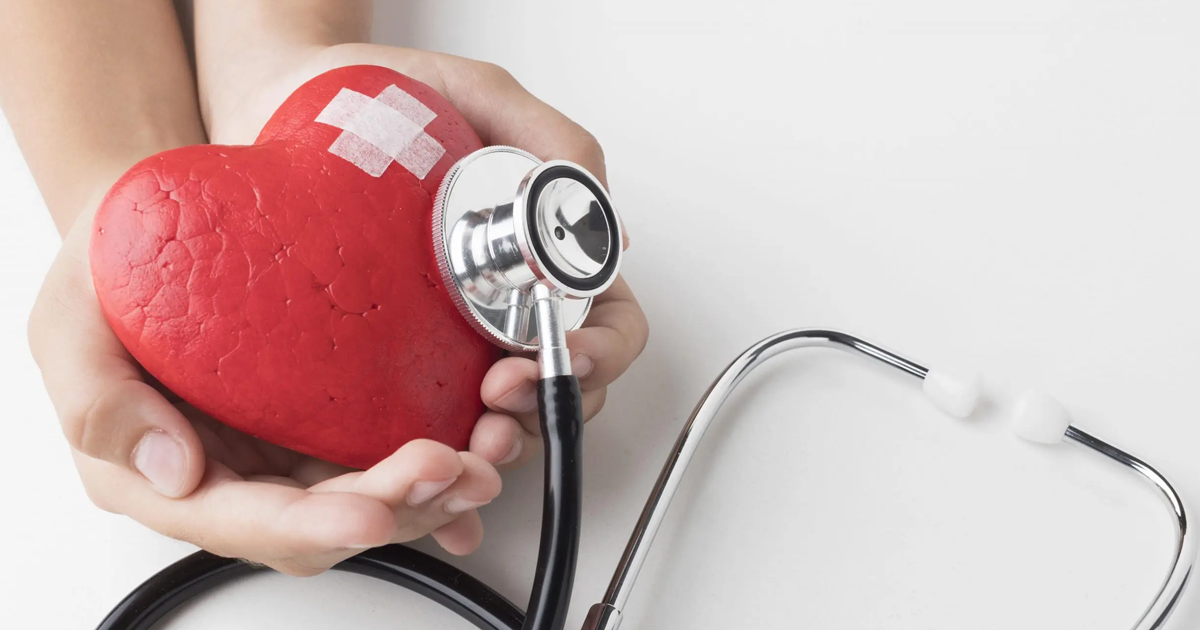
The study explains that, through machine learning models, it is possible to improve the diagnosis or prognosis of a disease, because it seeks to be based on evidence of the patient's physiology and condition, rather than on medical beliefs.
The authors explain that the usefulness of the model seeks to be effective in reducing hospital mortality and does not seek to predict the behavior of the disease.
Finally, the authors detail the following in the Discussion section: “The results of our experiments indicate machine learning models trained only on clinician-initiated administrative data can currently achieve performance close to models trained on more detailed, complete, EMR data”.
You can read the full paper, “Machine learning for patient risk stratification: standing on, or looking over, the shoulders of clinicians?” via the following link https://www.nature.com/articles/s41746-021-00426-3.