Nature published a new research based on Artificial Intelligence that, according to more than 50 thousand tests studied, would be able to predict COVID-19 by asking some basic questions.
The application of tests, the rapid isolation of infected patients, as well as the tracking of contacts are important measures to stop the transmission of the virus and thus reduce mortality by COVID-19. Equally important for health systems is rapid and efficient diagnosis, for which predictive models have been developed that can estimate the risk of infection. These are intended to facilitate the classification of patients in order to make better use of available resources.
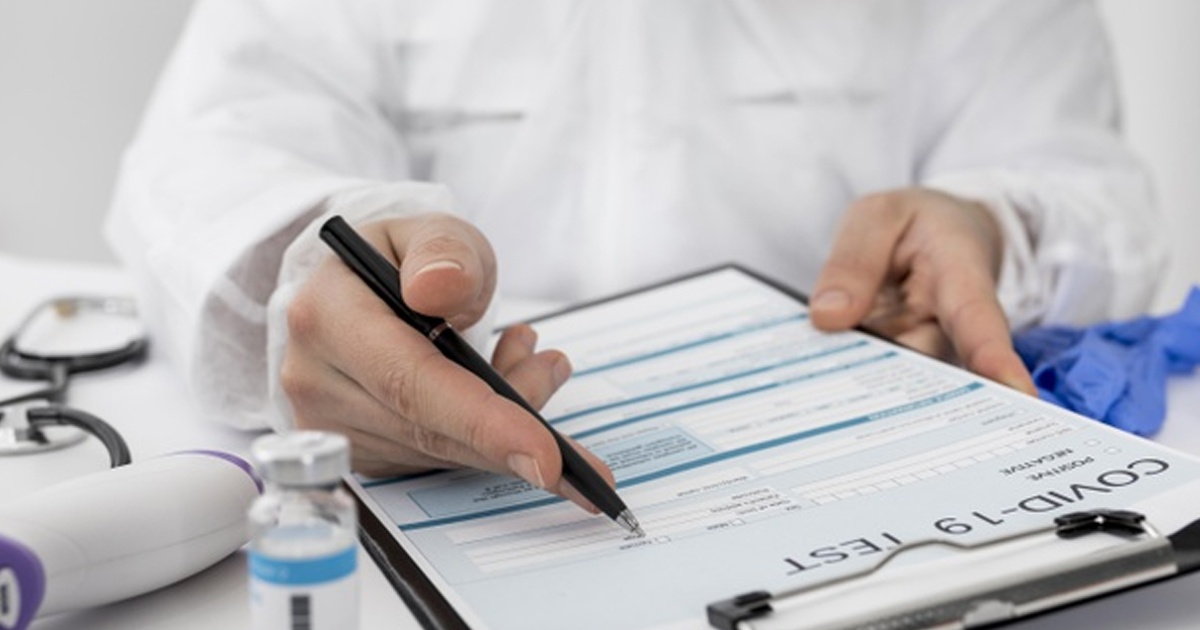
In the research published by Nature and its journal npj Digital Medicine entitled: “Machine learning-based prediction of COVID-19 diagnosis based on symptoms”, researchers planned an approach based on automatic learning with records of 51,831 people tested, of which 4,769 were positive. The data were obtained from the Ministry of Health of Israel, the researchers' place of origin.
The model predicted test results using eight characteristics: sex, age >60 years, contact with infected people, and the appearance of five symptoms. In this way, priority can be given to testing for COVID-19 when resources are limited.
The following is a list of the characteristics on which the prediction model was developed, with their category and response options:
- Basic information: Sex (male / female) and Age ≥60 years (true / false).
- Symptoms : Cough (true/false), Fever (true/false), Sore throat (true/false), Shortness of breath (true/false), Headache (true/false).
- Other Information: Known contact with an individual confirmed to have COVID-19 (true/false).
The researchers accepted some limitations of the model, due to available data on self-reported symptoms and contact of individuals with confirmed patients: “We showed that training and testing a model while filtering out symptoms of high bias in advance still achieved very high accuracy. We also note that all the symptoms were self-reported, and a negative value for a symptom might mean that the symptom was not reported. It is therefore important to assess the model’s performance in the circumstance that more values are unreported or missing rather than with negative values,” they explain in the discussion section.
However, in the research they explain that they carried out random tests to test the model and obtained important results, so they are confident about the model's effectiveness: To simulate a less biased condition, in our prospective test set, we randomly selected negative reports of all five symptoms at a time, and removed the negative values. When applied to these simulated test sets, the model still showed promising results, thus reinforcing our confidence in the model”.
To read the full article, click on the following link: https://www.nature.com/articles/s41746-020-00372-6#Abs1