Researchers at Chan Zuckerberg Biohub, a joint initiative of UC Berkeley, UC San Francisco and Stanford University, are working on a machine learning and cloud technology technique that can more accurately understand the number of uninsured COVID-19 cases.
Artificial Intelligence has played an increasingly relevant role for the medical sciences in recent years. In 2020, during the pandemic, various strategies have emerged from health research centers and health authorities in various countries to measure trends and developments in the epidemiological landscape.
This Chan Zuckerberg Biohub project is intended to be a tool to reduce the spread of COVID-19. “It is now well-known that asymptomatic infections are a common phenomenon in the spread of coronavirus. And it's very important to understand that phenomenon because, depending on how many asymptomatic infections there are, public health interventions might be different” said Dr. Lucy Li, data scientist at Chan Zuckerberg Biohub in an interview with HealthITAnalytics.
The same Li, in conjunction with researcher Patrick Ayscue also of the Chan Zuckerberg Biohub, published in June an article on the use of viral genomes to estimate undetected COVID-19 infections. “For disease outbreaks where you can detect every single infection, rapid testing and just a small amount of contact tracing is enough to get the epidemic under control. But for coronavirus, because there are so many asymptomatic infections out there, testing alone won't help control the pandemic”, she said.
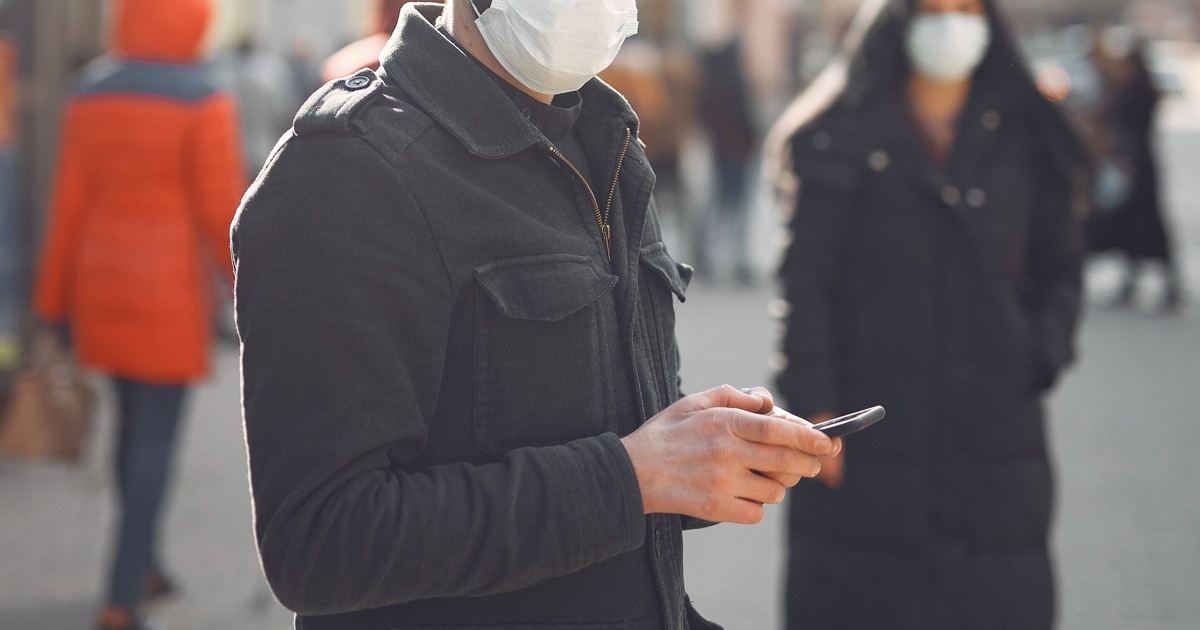
Through the exchange and increased availability of innovative data and tools, such as AI, through machine learning it will be possible to more accurately detect the behavior of contagions “The data I'm using are the viral genomes – the viral DNA. As the viral genomes spread through the population, they accumulate mutations. Generally, these mutations are not good or bad, they're just changes in the genome. Every time the virus is spread to a new person, it could accumulate new mutations. So, if we know how quickly the virus mutates, we can infer how many missing transmission links there were in between the observed genomes”, Li explained in an interview with the specialized website.
Through that type of data, it is how the model is trained as it can "simulate" different scenarios to know what is known about these viral genomes, in addition machine learning optimizes and streamlines these processes that previously required more time. “Before cloud computing became more common and these big computational resources became available, some of these analyses could take months to run. I've seen papers that were based on months of running a very complex model” explains the researcher, who at the same time recognizes the importance of applying new technologies to achieve better results. “But by having access to more computational resources in the cloud, we can shorten that time from months to days, because we're able to leverage much more memory and better parallelize our analysis.”
Scientists hope that this model can be adopted by health authorities in various countries. They also explained that in each place different results can be obtained, if an increase in under-registered cases is detected it may be necessary to increase the number of tests in the population, in addition also this type of research on a massive scale could help to know how close we are to the end of the pandemic. “By tracking how many people in the population have been infected by the virus or the number of undetected cases, we could get a sense of how far are we from eliminating this disease,” Li concluded.