The machine learning model, was trained through data from wearable devices such as smart watches or wearables. It was designed to achieve predictions from laboratory tests.
Study published in Nature entitled "Wearable sensors enable personalized predictions of clinical laboratory measurements". A study that explains the importance of measuring and collecting vital sign data, such as heart rate and body temperature. In this way it is possible to monitor and detect clinical conditions.
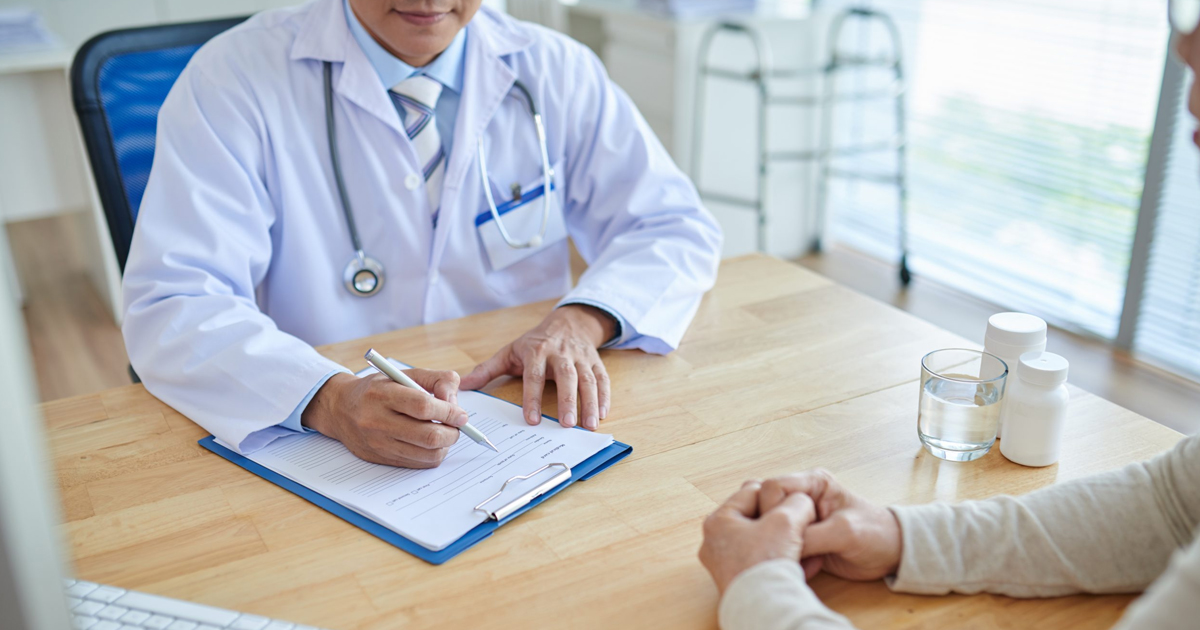
“Here we examined whether vital signs as measured by consumer wearable devices (that is, continuously monitored heart rate, body temperature, electrodermal activity and movement) can predict clinical laboratory test results using machine learning models, including random forest and Lasso models,” the study explains.
The results of the study showed that vital signs data obtained through wearablesprovide a more accurate description of resting heart rate than measurements taken in a clinic. In addition, the data collected can be used to predict various vital sign measurements that were obtained in a clinic.
“The length of time over which vital signs are monitored and the proximity of the monitoring period to the date of prediction play a critical role in the performance of the machine learning models,” the study explains.
The results showed that commercial, portable smart devices like wearables, can indeed be used for continuous, longitudinal assessment of physiological measures that could normally only be measured with laboratory tests.